구글 코랩(Colab)에서 VAE(Variational AutoEncoder) 모델 구현
감정분석 (Emotion Analysis) 연구를 위해 구글 Colaboratory 환경에서 VAE 모델을 학습시키고 예측하는 샘플 파이썬 코드를 작성하였습니다.
1. 라이브러리 설치 및 구글 드라이브 마운트
# 필요한 라이브러리를 설치합니다.
!pip install tensorflow
# Google Drive를 마운트합니다.
from google.colab import drive
drive.mount('/content/drive')
import os
os.environ["KERAS_BACKEND"] = "tensorflow"
import numpy as np
import pandas as pd
import tensorflow as tf
from tensorflow import keras
from tensorflow.keras import layers
from tensorflow.keras.preprocessing.text import Tokenizer
from tensorflow.keras.preprocessing.sequence import pad_sequences
2. Colab에 학습시킬 데이터 업로드
- 학습 데이터는 문장별로 17개의 감정중 1가지가 라벨링 되어 있습니다.
- 테스트 데이터셋 출처 : https://github.com/searle-j/KOTE
# 파일 경로 설정
file_path = '/content/drive/My Drive/emotion/emotion_dataset_korean.csv'
# 데이터 불러오기 (csv 파일에서 sentence와 label 컬럼이 있다고 가정합니다)
df = pd.read_csv(file_path, encoding='cp949')
sentences = df['sentence'].tolist()
labels = df['label'].tolist()
# 라벨 값 검토
print("Unique labels:", set(labels))
3. VAE 모델 구축
- 학습 데이터에 따라 아래 3가지 하이퍼파라미터 설정이 필요합니다.
- 잠재 공간 차원의 최적 갯수는 확인이 필요한데, 선행연구에서 제시한 8개로 우선 설정했습니다.
- 학습 시, 코렙 환경이 중도 종료될 경우를 대비하여 체크포인트 옵션을 추가했습니다.
# 하이퍼파라미터 설정
max_len = 40 # 최대 문장 길이, 단어
vocab_size = 10000 # 단어 집합 크기
embedding_dim = 16 # 임베딩 차원
# 토크나이저를 사용해 텍스트를 시퀀스로 변환
tokenizer = Tokenizer(num_words=vocab_size)
tokenizer.fit_on_texts(sentences)
sequences = tokenizer.texts_to_sequences(sentences)
padded_sequences = pad_sequences(sequences, maxlen=max_len, padding='post')
# 데이터셋 준비
data = np.array(padded_sequences)
class Sampling(layers.Layer):
"""Uses (z_mean, z_log_var) to sample z, the vector encoding a sentence."""
def __init__(self, **kwargs):
super().__init__(**kwargs)
self.seed = 1337
def call(self, inputs):
z_mean, z_log_var = inputs
batch = tf.shape(z_mean)[0]
dim = tf.shape(z_mean)[1]
epsilon = tf.random.normal(shape=(batch, dim), seed=self.seed)
return z_mean + tf.exp(0.5 * z_log_var) * epsilon
# 하이퍼파라미터
latent_dim = 8 # 잠재 공간의 차원
# 인코더 구축
encoder_inputs = keras.Input(shape=(max_len,))
x = layers.Embedding(input_dim=vocab_size, output_dim=embedding_dim, input_length=max_len)(encoder_inputs)
x = layers.LSTM(16, return_sequences=False)(x)
z_mean = layers.Dense(latent_dim, name="z_mean")(x)
z_log_var = layers.Dense(latent_dim, name="z_log_var")(x)
z = Sampling()([z_mean, z_log_var])
encoder = keras.Model(encoder_inputs, [z_mean, z_log_var, z], name="encoder")
encoder.summary()
# 디코더 구축
latent_inputs = keras.Input(shape=(latent_dim,))
x = layers.Dense(max_len * embedding_dim, activation="relu")(latent_inputs)
x = layers.Reshape((max_len, embedding_dim))(x)
decoder_outputs = layers.LSTM(embedding_dim, return_sequences=True)(x)
decoder_outputs = layers.TimeDistributed(layers.Dense(vocab_size, activation="softmax"))(decoder_outputs)
decoder = keras.Model(latent_inputs, decoder_outputs, name="decoder")
decoder.summary()
# VAE 모델 정의
class VAE(keras.Model):
def __init__(self, encoder, decoder, **kwargs):
super().__init__(**kwargs)
self.encoder = encoder
self.decoder = decoder
def train_step(self, data):
if isinstance(data, tuple):
data = data[0]
with tf.GradientTape() as tape:
z_mean, z_log_var, z = self.encoder(data)
reconstruction = self.decoder(z)
reconstruction_loss = tf.reduce_mean(
tf.reduce_sum(
keras.losses.sparse_categorical_crossentropy(data, reconstruction),
axis=1
)
)
kl_loss = -0.5 * tf.reduce_mean(
z_log_var - tf.square(z_mean) - tf.exp(z_log_var) + 1
)
total_loss = reconstruction_loss + kl_loss
grads = tape.gradient(total_loss, self.trainable_weights)
self.optimizer.apply_gradients(zip(grads, self.trainable_weights))
return {"loss": total_loss, "reconstruction_loss": reconstruction_loss, "kl_loss": kl_loss}
# VAE 모델 인스턴스화 및 컴파일
vae = VAE(encoder, decoder)
vae.compile(optimizer=keras.optimizers.Adam())
# 저장된 가중치 파일 로드
# 마지막에 저장된 가중치 파일의 경로를 제공해야 합니다.
latest_weights_filepath = '/content/drive/My Drive/emotion/checkpoints/vae_weights_05.weights.h5'
vae.load_weights(latest_weights_filepath)
# ModelCheckpoint 콜백 설정
checkpoint_filepath = '/content/drive/My Drive/emotion/checkpoints/vae_weights_{epoch:02d}.weights.h5'
checkpoint_callback = keras.callbacks.ModelCheckpoint(
filepath=checkpoint_filepath,
save_weights_only=True,
save_freq='epoch'
)
# 추가 학습
vae.fit(data, data, epochs=30, batch_size=2, callbacks=[checkpoint_callback])
# 최종 가중치 저장 (옵션)
vae.save_weights('/content/drive/My Drive/emotion/vae_model_final.weights.h5')
4. 필요 시, 가중치를 구글 클라우드에 저장
# 가중치 저장
vae.save_weights('/content/drive/My Drive/emotion/vae_model_5times_weights.h5')
# 가중치를 불러올 때
# 모델을 다시 정의한 후
#vae.load_weights('/content/drive/My Drive/emotion/vae_model_5times_weights.h5')
5. 노래 가사 감정 예측
- 노래 가사 문장별로 8개 잠재공간 차원별 가중치를 예측합니다.
- KPOP 노래 가사 파일
# 파일 경로 설정
file_path = '/content/drive/My Drive/emotion/kpop_lyrics.csv'
# CSV 파일 읽기
df = pd.read_csv(file_path, encoding='cp949')
new_sentences = df['lyric'].tolist()
new_sequences = tokenizer.texts_to_sequences(new_sentences)
new_padded_sequences = pad_sequences(new_sequences, maxlen=max_len, padding='post')
z_mean, z_log_var, z = encoder.predict(new_padded_sequences)
# 잠재 벡터를 데이터프레임에 추가
for i in range(latent_dim):
df[f'z_{i}'] = z[:, i]
# 수정된 데이터프레임을 구글 드라이브에 저장
output_file_path = '/content/drive/My Drive/emotion/kpop_lyrics_with_z.csv'
df.to_csv(output_file_path, index=False, encoding='cp949')
print(f"Data with latent vectors saved to {output_file_path}")
# 디코더를 사용해 재구성된 문장 생성
#reconstructed_sentences = decoder.predict(z)
#print("Reconstructed sentences:", reconstructed_sentences)
6. 예측 결과
- 가사 별로 8가지 감정으로 예측한 결과를 확인할 수 있습니다.
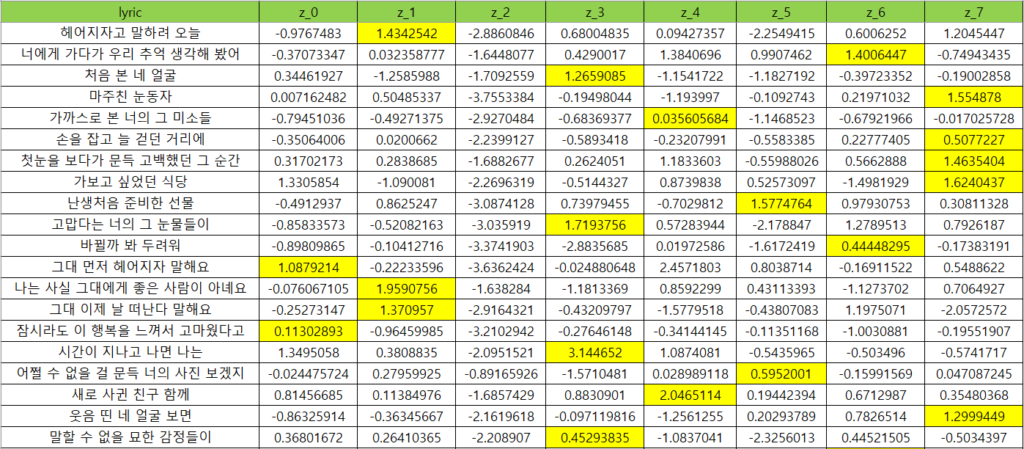
Subscribe
Login
0 Comments
Oldest